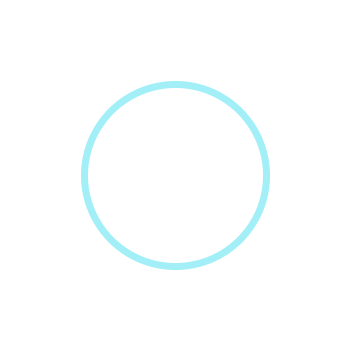
Photo by Choong Deng Xiang on Unsplash
A Story of a Data Scientist
Let me introduce you to Sarah, a talented and passionate data scientist, who just landed her dream job at GreenEnv, a large company that makes eco-friendly cleaning products. GreenEnv has tons of data on customers, products, and other areas of the business. They hired Sarah to unlock the hidden potential within this data, uncovering market trends, competitive advantages, and more.
Her first task: analyze customer demographics and buying habits to create targeted marketing campaigns. Confident in her abilities and excited to apply data science methods, Sarah dived into the customer database. But her initial excitement quickly faded. The data was a mess — inconsistent formatting, misspelled names, and duplicate entries everywhere. Data quality was terrible. There were variations of names like “Jhon Smith” and “Micheal Brown” alongside entries like “Jhonn Smtih” and “Michealw Brown.” Emails had extra spaces and even typos like “gnail.com” instead of “gmail.com.” along with many other inaccuracies. Sarah realized the hard job ahead of her — data cleaning.
Inconsistent formatting, missing values, and duplicates would lead to skewed results, giving an inaccurate picture of GreenEnv’s customer base. Days turned into weeks as Sarah tirelessly cleaned the data, fixing inconsistencies, filling in gaps, and eliminating duplicates. It was a tedious process, but essential to ensure her analysis was built on a solid foundation.
Who cares about data quality?Every year, poor data quality costs organizations an average of $12.9 million. [1]
Thankfully, after weeks of cleaning and organizing this messy data, Sarah was able to get the job done…or at least for this part..
Her next challenge came when she ventured into product data, aiming to identify top-selling items and recommend future opportunities. However, she encountered a different problem — a complete lack of metadata. Product descriptions were absent, and categories were ambiguous. Basically, there wasn’t enough data to help Sarah to understand the product’s data. Sarah realized the importance of metadata management — structured information about the data itself. Without it, understanding and analyzing the data was almost impossible.
Research Shows Most Data Has InaccuraciesResearch by Experian reveals that businesses believe around 29% of their data is inaccurate in some way. [2]
Frustrated but determined, Sarah reached out to different departments to piece together information about the products. She discovered that each department used its own internal jargon and classification systems. Marketing and sales refer to the same cleaning product with different names.
As Sarah delved deeper, she found that datasets were kept in separate applications by different departments, outdated storage systems struggling to handle the growing volume of data, and Sarah had to wait for a long time for her queries to be executed. Sarah noticed also there are no clear rules on who can access what data and under what terms, without centralized control and proper access controls, the risk of unauthorized access to sensitive information increases, potentially leading to data breaches and compliance violations. The lack of data governance, a set of rules and procedures for managing data, was evident.
Data Breaches Can Be CostlyAccording to the Ponemon Institute, the average cost of a data breach in 2023 is $4.45 million globally, an all-time high record, with costs varying by industry and location. [3]
Each of the above issues and hurdles in Sarah’s story highlighted the interconnectedness of many pillars — data quality, metadata management, and data governance all played a crucial role in accessing and utilizing valuable insights at GreenEnv.
Sarah’s journey is a common one for data scientists and analysts. Many organizations have massive amounts of data, and everyone knows the saying: “Data is the new electricity.” Every organization wants to make the most of their data, as it’s a very valuable asset. But most people mistakenly (and practically) believe that simply hiring a data analyst or data scientist is enough to unlock this value. There are many pillars to getting the most value from data, and organizations need to account for and pay attention to these. The keyword here is data management.
Did you know..86% of organizations say they believe investing in data management directly impacts their business growth[4]
What Exactly is Data Management?
Generally speaking, data management is the overall practice of handling the organization’s data. from acquiring and storing the data to processing, securing, and analyzing it. The goal is to ensure the data is accessible, usable, accurate, reliable, and of high quality to achieve the state of data-informed organization and ultimately achieve our organizational objectives.
The Pillars of Data Management
There are several key dimensions that work together to achieve successful data utilization, and while these pillars might be slightly different from one framework to another, the underlying concepts are the same.
In addition to data security and data integration as pillars we also have:
Data Quality: This ensures the data is accurate, consistent, complete, and timely. It involves identifying and fixing errors, managing missing values, and establishing data cleaning and validation processes.Metadata Management: This focuses on organizing and cataloging information about the data itself.Data Governance: This establishes the framework and rules for managing data assets throughout their lifecycle. It involves setting policies, and processes, and assigning roles to ensure data accuracy, security, and compliance with regulations.Data Architecture: This defines the structure and organization of data assets. It includes defining data models, storage solutions, and data flows, ensuring efficient data storage, retrieval, and utilization.Data Lifecycle Management: This focuses on managing data throughout its entire lifespan, from creation to archiving or deletion. It involves implementing processes for data capture, storage, transformation, use, and disposal.
You can notice that these dimensions are interconnected and also closely related. Addressing weaknesses in one area can often impact others. Some frameworks have been developed to address the relationship between the different dimensions of data management and how they interact and affect each other.
Data Management Frameworks: The Aiken’s Pyramid
Aiken’s Pyramid: Image by Author
Many established frameworks like DAMA-DMBOK2, IGF, and EDM offer structured guidance, standardized terminology, and maturity assessments for data management
One conceptual framework worth mentioning here, and the one I like the most, is Aiken’s pyramid of Data Management. It outlines the different stages of data management processes. Developed by Robert Aiken, a data management pioneer, this framework describes the situation in which many organizations find themselves. In trying to leverage the full potential of their data, many organizations go through a similar progression of steps:
Phase 1: This focuses on establishing the basic building blocks, like data modeling, storage solutions, and security measures.Phase 2: As data usage increases, this level addresses challenges arising from poor data quality and activities like metadata management and data architecture.Phase 3: The previous activities from Phase 2 require data governance. data governance also enables activities like document and content management, reference and master data management, data warehousing, and business intelligence, all in turn allowing for advanced analytics in Phase 4.Phase 4: This is the stage where the organization truly unlock the full potential of their data. Here, organizations leverage high-quality data for advanced analytics and data science and extract valuable insights to inform decision-making.
The Aiken Pyramid helps organizations understand how data management activities interconnect, how each one builds on the others, and how to prioritize their efforts for effective data utilization.
My Reflections and Takeaways on Data Management Best Practices
Reflecting on learning and experience with data management(although I’m not a data management expert XD), I’ve come to appreciate and favor the following points regarding data management and its best practices, especially if we are focusing on data quality.
There’s no one-size-fits-all solution to data management. While frameworks exist to guide organizations towards data management maturity, the full process remains unique for each entity. Each organization prioritizes different aspects of data management and faces distinct challenges.My approach would be to start simple. Apply data management best practices or enhancements to a targeted portion of the organization’s data, focusing on what matters most. This allows for gradual growth in maturity, eventually encompassing all data. This phased approach can be very beneficial for dimensions like data quality and metadata management.If a process consistently generates bad data, even the best efforts in other areas of data management won’t prevent it. These processes can be technical or non-technical. A proactive approach is crucial here.
– For example, a non-technical process that generates bad data might involve database creation by developers who solely focus on the technical aspects. There might be a lack of documentation or column descriptions for instance. A good practice in my opinion would be to engage data analysts and other relevant stakeholders in the design process to ensure adherence to data management best practices. The data management team can decide if we would go forward with a certain application design or not.
– An application’s design can also be a technical process for bad data generation. A well-designed application should enforce data quality proactively during data entry. For instance, instead of a text box for entering gender, a dropdown menu could be used. Another example might be predefining email types, where the user only needs to add their username before automatically receiving “@gmail.com” or another domain extension.Standardization is key: Inconsistency in data can be a nightmare. Imagine customer names stored differently across departments, dates in conflicting formats, or teams using their own abbreviations. but more than that within a single organization or a company there might be different processes that generate the same type of data, and different data collection tools. Standardization combats this chaos by establishing common formats, definitions, and processes for data handling. This ensures data quality, simplifies integration across applications, fosters collaboration through a shared data language, and boosts efficiency by streamlining data workflows. This process is also iterative and agile, the organization can gradually achieve more levels of maturity in it. This one can also be part of the previous data management process of validation of applications that generate data: adherence to standards. i.e. any application to be approved should comply to the standards first.Finally, data management is a comprehensive process that requires collaboration across different teams within the organization, with the need to define the data management strategy and align it with the business or institute’s objectives and strategies. This would typically start with assessing the current and desired data management maturity levels, analyzing the gap, prioritizing data management tasks, and remaining agile. The process is iterative, and clear solutions rarely exist in advance.
Data Management Professional Career
There are many data management certifications out there you might consider. The best choice depends on your specific goals and experience but here are a few ones I came across:
Certified Data Management Professional (CDMP): Offered by the Data Management Association (DAMA) International, this covers a wide range of topics, from data governance and quality to modeling.Certified Information Management Professional (CIMP): This program, offered by the Institute for Information Management (IIM), focuses on information management disciplines like governance, quality, security, and more.Data Governance and Stewardship Professional (DGSP): This certification, from the Data Governance Institute, focuses on the skills needed to develop and implement a data governance program, along with ensuring data quality, compliance with regulations, and so on.Certified Clinical Data Manager (CCDM): This one, offered by the Society for Clinical Data Management (SCDM), is for professionals in clinical research who manage data collected during trials.
Ultimately the choice of which one is worth the time and effort requires a more in-depth analysis of the credibility, content, and purpose of each certification.
Looking Ahead: Data Management Tools
Image by author
As you would guess, there is an endless number of tools available to address different aspects of data management, and it would be impractical to talk about all these tools, each tool would have pros and cons, and situations where it might be effective, or specific data management dimensions where it would be helpful.
In my next article, I will delve deeper into two open-source tools for data management, DataHub and Great Expectations(GX), and provide a step-by-step guide on how to integrate them to create a robust, cost-effective, scalable, and unified environment for data discovery, metadata management, data quality, data governance, data lineage, and impact analysis.
Conclusion
Data is arguably an organization’s most valuable asset these days. However, many still lack proper data management, limiting their ability to leverage its true potential. Issues like data quality, governance, security, and metadata management are all central pillars to get the most value out of your organization’s data.
Remember Sarah’s story at the beginning? Hiring a data scientist isn’t enough. They often spend a significant amount of time cleaning and organizing messy data before they can even begin analysis. Thankfully, frameworks like the Aiken Pyramid can guide organizations on their data management journey, and help in communicating data management initiatives across the different teams and different stakeholders easily and regardless of their technical level.
Thanks for reading all the way here! I hope you found this informative and enjoyable. Please feel free to point out any mistakes or share your thoughts — your feedback is always appreciated.
References
Gartner. (2021). “How to Improve Your Data Quality.” Smarter With Gartner, Gartner”Experian. (2019). “Global Data Management Research Report.”Ponemon Institute. (2023). “Cost of a Data Breach Report.”Experian. (2021), “Data experience The data-driven strategy behind business growth report”
Further Readings
Books
Data Management Body of Knowledge (DAMA-DMBOK): The DAMA-DMBOK 2nd edition, this one serves as a comprehensive guide to data management practices and principles. It provides a detailed overview of various aspects of data management. [my favorite reference for data management!]“Data Governance: How to Design, Deploy and Sustain an Effective Data Governance Program” by John Ladley.“The Case for the Chief Data Officer: Recasting the C-Suite to Leverage Your Most Valuable Asset”, by Peter Aiken, Michael M. Gorman“Data Management for Researchers: Organize, Maintain and Share Your Data for Research Success” by Kristin Briney.
Online Resources:
Data Management Association (DAMA) International: DAMA International offers a wealth of resources on data management, including articles, webinars, and whitepapers. Their website is a valuable resource for both beginners and experienced professionals.The Data Administration Newsletter: TDAN.com is an online publication dedicated to data management and data administration topics.CDMP Study Group on Facebook: Here you can find many data practitioners and others who are interested in the CDMP exam, you can ask questions, seek a study partner, or join them in their regular webinars and discussions about data management based on the CDMP topics. personally, this one is one of my favorites, thanks to Nicole Janeway Bills for her efforts in this group.
The Many Pillars of Getting the Most Value From Your Organization’s Data was originally published in Towards Data Science on Medium, where people are continuing the conversation by highlighting and responding to this story.