Comparing model-free and model-based RL methods on a dynamic grid world Photo by Saffu on Unsplash Recently, Reinforcement Learning (RL) algorithms have received a lot of traction by solving research problems such as protein folding, reaching a superhuman level in drone racing,
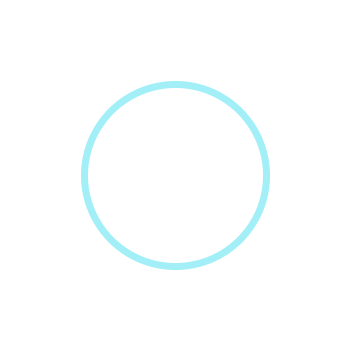
Recent Comments